Significant Increase in Power Output of Thermoelectric Materials Using Machine Learning
—New Approach to Chemical Composition Optimization May Expedite Practical Use of Thermoelectric Materials Composed of Abundant Elements—
2019.03.19
National Institute for Materials Science
Graduate School of Frontier Sciences, University of Tokyo
Japan Science and Technology Agency
A NIMS-University of Tokyo research group has succeeded in greatly enhancing the power output of thermoelectric materials composed of abundant elements—aluminum, iron, and silicon—using machine learning scheme. Machine learning prediction enabled the group to discover optimum chemical compositions, which the conventional experimental approach had failed to detect, resulting in a 40% increase in power output. Machine learning may dramatically accelerate R&D of thermoelectric materials composed only of abundant elements toward practical use.
Abstract
- A NIMS-University of Tokyo research group has succeeded in greatly enhancing the power output of thermoelectric materials composed of abundant elements—aluminum, iron, and silicon—using machine learning scheme. Machine learning prediction enabled the group to discover optimum chemical compositions, which the conventional experimental approach had failed to detect, resulting in a 40% increase in power output. Machine learning may dramatically accelerate R&D of thermoelectric materials composed only of abundant elements toward practical use.
- The anticipated “IoT society” in which various devices will be connected via the internet is expected to increase demand for sensors dramatically. Thermoelectric materials are key to the emergence of IoT because they are capable of directly converting heat into electricity and are thus potentially applicable to the development of small, autonomous power sources suitable for sensors and wearable devices. Thermoelectric materials have some outstanding characteristics. They can generate electricity using only small temperature differences and can be miniaturized, and they require no maintenance. However, thermoelectric materials have several disadvantages; materials contain rare or toxic chemical elements, and its operating temperature range is narrow, requiring the production of different conduction types of thermoelectric materials for the different environments in which they are used. NIMS has been striving to develop thermoelectric materials composed only of nontoxic and relatively abundant chemical elements for broader spread, and recently succeeded in developing a novel thermoelectric material composed of aluminum (Al), iron (Fe), and silicon (Si) capable of producing power output using a temperature range between room temperature and 200°C (PCT/JP2018/032031).
- This joint research group further optimized the composition of the Al-Fe-Si-based thermoelectric material developed by NIMS using a new technique which combined machine learning (Bayesian optimization) with an experimental approach, enabling the power generation using a wider temperature range up to 400°C. After learning a limited amount of experimental data (chemical compositions, power factor, and temperatures), the machine learning prediction was able to provide a suggested chemical composition to enhance the power output property in a medium- temperature range (200-400°C). These predicted compositions deviated from the composition range investigated by the conventional experimental approach. The group actually synthesized the Al-Fe-Si-based material with the predicted composition and found that its power output was 40% higher than that of the initial composition. In addition, the power output performance of the material decreased when its composition slightly deviated, indicating that the predicted composition was optimum one.
- These results demonstrated that machine learning technique is a valuable tool for the development of thermoelectric materials and its use may lead to the discovery of novel materials with more complex compositions which would be difficult to identify using by an experimental approach. The present results may also further facilitate R&D on thermoelectric power generation applicable for autonomous power sources of a variety of sensors in IoT society.
- This research project was carried out by Yoshiki Takagiwa (Senior Researcher, Thermal Management and Thermoelectric Materials Group, Center for Materials Research by Information Integration [CMI2], Research and Services Division of Materials Data and Integrated System [MaDIS], NIMS), Zhufeng Hou (Special Researcher, Data Platform Group, CMI2, MaDIS, NIMS), Yoshikazu Shinohara (Thermal Management and Thermoelectric Materials Group, CMI2, NIMS; also Leader of the Thermoelectric Materials Group, Center for Green Research on Energy and Environmental Materials, NIMS), Yibin Xu (Platform Director, Data Platform Group, NIMS) and a research group led by Koji Tsuda (Professor, Graduate School of Frontier Sciences, University of Tokyo).
This project was conducted as part of the “Materials Research by Information Integration” Initiative (MI2I) supported by the Japan Science and Technology Agency (JST)’s Support Program for Starting Up Innovation Hub implemented at the MaDIS division of NIMS. - This research was published in the online version of ACS Applied Materials & Interfaces at 11:00 am on March 18, 2019, Eastern Standard Time.
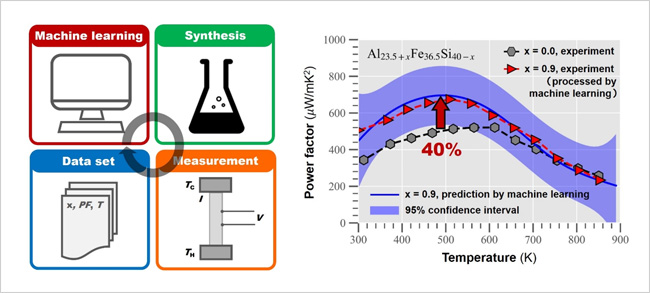
Figure.
(Left) The flow of steps in machine learning-integrated materials R&D.
(Right) The machine learning approach led to an approximately 40% increase in power factor in the medium temperature range.
Related files
- Center for Materials Research by Information Integration
Contacts
(Regarding this research)
-
Yoshiki Takagiwa
Senior Researcher, Thermal Management and Thermoelectric Materials Group,
Center for Materials Research by Information Integration [CMI2], Research and Services Division of Materials Data and Integrated System [MaDIS],
National Institute for Materials Science
Tel: +81-29-859-2811
E-Mail: TAKAGIWA.Yoshiki=nims.go.jp
(Please change "=" to "@") -
Koji Tsuda
Professor, Department of Computational Biology and Medical Sciences
Graduate School of Frontier Sciences
University of Tokyo
5-1-5-CB02 Kashiwanoha, Kashiwa, Chiba 277-8561, Japan
Tel: +81-4-7136-3983
E-Mail: tsuda=k.u-tokyo.ac.jp
(Please change "=" to "@")
(For general inquiries)
-
Public Relations Office
National Institute for Materials Sciences
Tel: +81-29-859-2026
Fax: +81-29-859-2017
E-Mail: pressrelease=ml.nims.go.jp
(Please change "=" to "@") -
Public Relations Office
Graduate School of Frontier Sciences,
University of Tokyo
5-1-5 Kashiwanoha, Kashiwa, Chiba 277-8561, Japan
Tel: +81-4-7136-5450
E-Mail: taguchi.yumie=edu.k.u-tokyo.ac.jp
(Please change "=" to "@") -
Public Relations Division
Japan Science and Technology Agency
5-3 Yonbancho, Chiyoda-ku, Tokyo 102-8666, Japan
Tel: +81-3-5214-8404
Fax: +81-3-5214-8432
E-Mail: jstkoho=jst.go.jp
(Please change "=" to "@")
(Regarding JST projects)
-
COI Group
Department of Innovation Platform
Japan Science and Technology Agency
K's Gobancho, 7 Gobancho, Chiyoda-ku, Tokyo 102-0076, Japan
Tel: +81-3-6267-4752
Fax: +81-3-5214-8496
E-Mail: ihub=jst.go.jp
(Please change "=" to "@")