Accelerating Thin Film Fabrication Processes Using Machine Learning
—Technique May Be Used to Reduce Materials Development Cost by Greatly Shortening the Trial-and-Error Process without Relying on External Data—
2020.10.21
National Institute for Materials Science (NIMS)
University of Tokyo
A NIMS-University of Tokyo research group has developed a machine learning technique which can be used to expedite the process of determining optimum conditions for the fabrication of high-quality thin films by reducing the number of material samples needed to be evaluated by up to 90% compared to currently available thin film fabrication methods.
Abstract
- A NIMS-University of Tokyo research group has developed a machine learning technique which can be used to expedite the process of determining optimum conditions for the fabrication of high-quality thin films by reducing the number of material samples needed to be evaluated by up to 90% compared to currently available thin film fabrication methods. The technique may be used to reduce the cost of developing many different types of thin film materials.
- Nanometer-scale-thick film materials are fabricated using various types of materials for different purposes. Thin film R&D has been conducted at different levels, ranging from basic research to industrial application. Fabrication of high-quality, reproducible thin films requires optimization of many parameters (e.g., temperature and raw material supply rate). To identify the combination of optimal parameter values, a large number of samples—sometimes hundreds of them—need to be created under different parameter settings and evaluated. To facilitate this process, it is desirable to develop techniques capable of determining optimal thin film fabrication conditions without needing to evaluate so many samples.
- This research group developed a machine learning technique, which: when combined with thin film fabrication processes, can determine optimum fabrication parameters by evaluating a substantially lower number of material samples than currently available methods. In this technique, the machine learning model is initially trained using data from evaluation of the first several thin film samples fabricated under different parameter settings. The trained model then estimates optimum parameters to be used for the subsequent thin film fabrications. Several repetitions of training sessions enable the model to accurately estimate optimum fabrication conditions without requiring many additional samples to be evaluated. The group applied this technique to the fabrication of titanium nitride (TiN) thin films whose superconducting transition temperatures and other physical properties are influenced by their crystalline properties. After repeating thin film fabrication experiments 11 times, including the initial six experiments to generate training data, the group succeeded in determining the optimum parameter combinations for the fabrication of TiN thin films that achieve the highest superconducting transition temperatures.
- The use of this technique may reduce the cost of thin film development by eliminating the need to rely on external databases. In addition, the technique may be used to speedily fabricate various types of thin films under optimum conditions in a less labor-intensive manner, potentially benefiting thin film research efforts at different levels, ranging from basic research to the development of efficient manufacturing processes.
- This project was carried out by a research group led by Isao Ohkubo (Senior Researcher, International Center for Materials Nanoarchitectonics (MANA), NIMS), Takashi Aizawa (Chief Researcher, MANA, NIMS), Takao Mori (Group Leader, MANA, NIMS), Zhufeng Hou (Center for Materials Research by Information Integration (CMI2), NIMS; currently affiliated with the Chinese Academy of Sciences), Toyohiro Chikyow (Senior Scientist with Special Missions, CMI2, NIMS), Koji Tsuda (Professor, Graduate School of Frontier Sciences, University of Tokyo; also a member of the CMI2, NIMS), Mikk Lippmaa (Professor, Institute for Solid State Physics (ISSP), University of Tokyo) and Jiyeon N. Lee (PhD student, ISSP, University of Tokyo). This project was conducted in conjunction with other research projects, including 1) a project titled “Novel materials exploration driven by ab initio calculations and informatics” (research director: Isao Ohkubo, project number: JPMJPR15N1) under the category of the JST Strategic Basic Research Program PRESTO titled “Advanced materials informatics through comprehensive integration among theoretical, experimental, computational and data-centric sciences” (research supervisor: Shinji Tsuneyuki), 2) a project titled “Materials research by information integration initiative (MI2I)” sponsored by the JST Support Program for Starting Up Innovation Hub, 3) a project titled “Development of materials design workflow and data library for materials foundry" (project leader: Toyohiro Chikyow, project number: JPMJMI18G5)) under the JST-Mirai Program category “Common platform technology, facilities and equipment” and 4) a project titled “Utilizing magnetism to develop high performance thermoelectric materials and devices” (project leader: Takao Mori, project number: JPMJMI19A1) supported by the JST-Mirai Program.
- This research was published in the online version of Materials Today Physics on September 28, 2020.
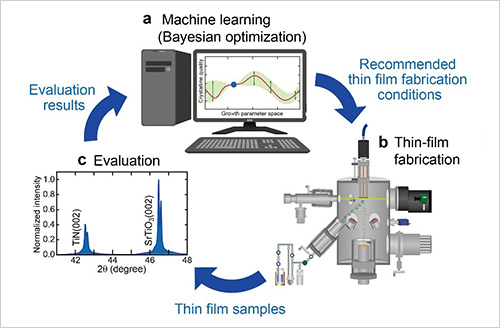
Figure. Schematic illustration of a machine-learning-integrated closed-loop process to optimize thin film fabrication parameters
Related files
- MANA
Contact information
(Regarding this research)
-
Isao Ohkubo
Senior Researcher
Thermal Energy Materials Group
International Center for Materials Nanoarchitectonics
National Institute for Materials Science
Tel: +81-29-860-4761
E-Mail: OHKUBO.Isao=nims.go.jp
(Please change "=" to "@")
(General information)
-
Public Relations Office
National Institute for Materials Science
Tel: +81-29-859-2026
Fax: +81-29-859-2017
E-Mail: pressrelease=ml.nims.go.jp
(Please change "=" to "@") -
Public Relations Office
Institute for Solid State Physics
University of Tokyo
Tel: +81-4-7136-3207
E-Mail: press=issp.u-tokyo.ac.jp
(Please change "=" to "@") -
Public Relations Office
Graduate School of Frontier Sciences
University of Tokyo
Tel: +81-4-7136-5450
E-Mail: press=k.u-tokyo.ac.jp
(Please change "=" to "@")
Same Keywords
-
Significant Increase in Power Output of Thermoelectric Materials Using Machine Learning
2019.03.19
-
Hundreds-fold Electrochemical Measurement Output Brings Data Science to Reveal Microbial Electricity Generation Mechanisms
2022.10.25
-
Success in Efficient Fabrication of High-Performance Neodymium Magnets Using Machine Learning
2021.11.15