Successful Prediction of Alcohol Contents through Smells of Liquors
—Combination of a Sensitive / Compact Sensor and Machine Learning Enables Quantification of Various Odor Information—
2017.06.20
National Institute for Materials Science(NIMS)
A NIMS research group has succeeded in accurately predicting the alcohol contents of liquid samples through their smells by combining a Membrane-type Surface stress Sensor (MSS) with functional nanoparticles and machine learning technique.
(“Data-driven nanomechanical sensing: specific information extraction from a complex system”; Kota Shiba, Ryo Tamura, Gaku Imamura, and Genki Yoshikawa; Scientific Reports 7, Article number: 3661 (2017), doi:10.1038/s41598-017-03875-7)
Abstract
- A NIMS research group consisting of Kota Shiba (Researcher, Nanomechanical Sensors Group, WPI-MANA), Ryo Tamura (Researcher, First-Principles Simulation Group, WPI-MANA), Gaku Imamura (ICYS-NAMIKI Researcher, International Center for Young Scientists) and Genki Yoshikawa (Group Leader of the Nanomechanical Sensors Group, WPI-MANA) has succeeded in predicting the alcohol contents of liquid samples through their smells by combining a Membrane-type Surface stress Sensor (MSS) with functional nanoparticles and machine learning technique. This approach allows simple and accurate quantification of specific information extracted from complex smells. The technique potentially has diverse applications, such as the quantification of fruit maturity and human health conditions.
- Smells are known to be a complex mixture of gases generally composed of hundreds to thousands of different types of compounds. Because of this complexity, extraction and quantification of specific information of a smell, such as composition and concentration of gas components, usually requires separation and analysis of individual gas components using relatively large equipment, such as gas chromatographs.
- The research team recently developed a new technique to quantify specific information from smells through the integration of three technological components: MSS, functional nanoparticles and machine learning. As a demonstration of the new technique, the team accurately quantified the alcohol contents of liquid samples. Specifically, the team first prepared multiple MSS coated with different types of sensing materials which absorb odor molecules, and then measured the smells of various types of alcohol, such as beer and vodka, recording the electrical signal patterns specific to each type of alcohol. The team constructed a prediction model by training the datasets of electrical signal patterns and the alcohol contents of liquid samples using machine learning. The team then optimized the system to increase the prediction accuracy by selecting more suitable sensing materials, identifying effective signal features, and optimizing feature selection, based on the information obtained from machine learning. Through such mutual optimization between hardware (the MSS and sensing materials) and software (the prediction model), the team succeeded in accurately quantifying the alcohol contents of liquors (red wine, spirits distilled from sweet potatoes and whisky) that were not used to train the prediction model.
- In addition to alcohol content, the new technique may also allow the simple and accurate extraction of various information. For example, the technique may be used to predict fruit maturity and human health conditions by correlating numerical values of such conditions with smells. Based on the presented results, the team plans to pursue various consumer-level and industrial applications of the technique, such as food and agricultural product quality management, health examinations and environmental monitoring.
- This research was supported by the JST CREST program (JPMJCR1665), a Grant-in-Aid for Scientific Research (B) (Research Report Number: 15H03588), a Grant-in-Aid for Young Scientists (B) (Research Project Number: 16K21602), and the "Materials Research by Information Integration" Initiative sponsored by the JST’s Support Program for Starting up Innovation Hub.
- This study was published in the online version of Scientific Reports on June 16, 2017 (local time).
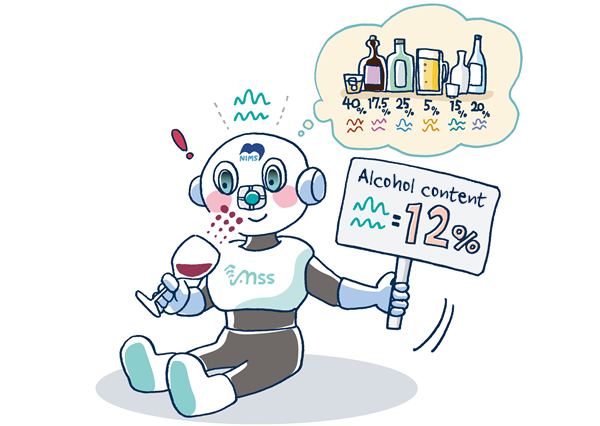
Related files
- MANA
- ICYS
Contacts
(Regarding this research)
-
Kota Shiba
Researcher, Nanomechanical Sensors Group
International Center for Materials Nanoarchitectonics (WPI-MANA)
National Institute for Materials Science
Tel: +81-29-860-4603
E-Mail: SHIBA.Kota=nims.go.jp
(Please change "=" to "@") -
Ryo Tamura
Researcher, First-Principles Simulation Group
International Center for Materials Nanoarchitectonics (WPI-MANA)
National Institute for Materials Science
Tel: +81-29-860-4948
E-Mail: TAMURA.Ryo=nims.go.jp
(Please change "=" to "@")
(For general inquiries)
-
Public Relations Office
National Institute for Materials Science
Tel: +81-29-859-2026
Fax: +81-29-859-2017
E-Mail: pressrelease=ml.nims.go.jp
(Please change "=" to "@")