Press Release 2021
Digitalization and Visualization of Odors Using an Odor Sensor and Machine Learning
—Technique Is Capable of Selecting “Quasi-Primary Odors” Out of a Dozen Odors—
NIMS has developed a technique which combines an odor sensor and machine learning that is capable of selecting reference odors out of a dozen samples of different odors.
“Determination of quasi-primary odors by endpoint detection”
Hanxiao Xu, Koki Kitai, Kosuke Minami, Makito Nakatsu, Genki Yoshikawa, Koji Tsuda, Kota Shiba, and Ryo Tamura;
Journal: Scientific Reports 11, 12070 (2021) [June 9, 2021 (JST)];
DOI : 10.1038/s41598-021-91210-6)
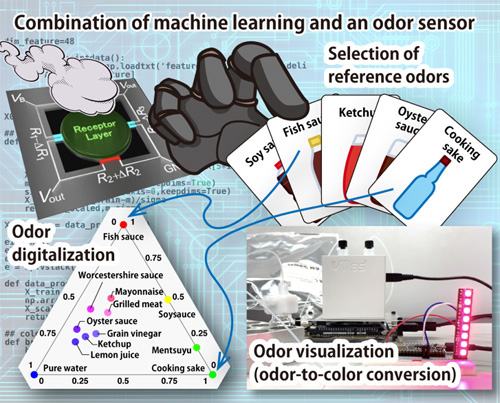
Figure. Quasi-primary odor determination using a combination of machine learning and an odor sensor. If quasi-primary odors are selected by the proposed method, all odors are digitalized in terms of the quasi-primary odor mixture ratios. In addition, quantified odors can be visualized using colors.
Abstract
Scientific research to define primary odors has long been conducted in an effort to establish a concept comparable to the three primary colors of light and the five primary tastes. However, this effort has proven difficult, mainly because human olfactory receptors, which convert olfactory stimuli into electrical signals, are enormously more diverse (approximately 400 types) than visual and taste receptors.
This research team took the approach of determining reference odors (“quasi-primary odors”) out of a limited number of odor samples rather than determining true primary odors out of a full range of known odors. Specifically, samples of different odors were collected and measured using a multichannel membrane-type surface stress sensor (MSS)—a highly sensitive, compact odor sensor developed primarily by NIMS. The data was then analyzed using a machine learning technique, and the few odors that differed most significantly from other odors sampled were designated as reference odors (i.e., quasi-primary odors). The team was able to digitalize all odors sampled in terms of the quasi-primary odor mixture ratios. For example, when 12 liquid samples were analyzed (pure water and 11 different seasonings), three odor sources (fish sauce, cooking sake and pure water) were selected as quasi-primary odors. The team was then able to quantify all of the other seasonings, including soy sauce and grilled meat sauce, in terms of the mixture ratios of these quasi-primary odors.
Quantified odors can be visualized using colors. For example, assigning individual quasi-primary odors different colors enables visualization of various odors as different mixtures of these colors. To actually demonstrate this, the team developed a device capable of chromatically displaying odors as they are detected by the MSS.
This project was carried out by Ryo Tamura (Senior Researcher, International Center for Materials Nanoarchitectonics (WPI-MANA), NIMS), Kota Shiba (Senior Researcher, Research Center for Functional Materials (RCFM), NIMS), Kosuke Minami (Research Fellow, International Center for Young Scientists, NIMS), Genki Yoshikawa (Group Leader, RCFM, NIMS), Koji Tsuda (Invited Researcher, Research and Services Division of Materials Data and Integrated System (MaDIS), NIMS), Hanxiao Xu (Trainee, MaDIS, NIMS at the time of this project), Koki Kitai (Trainee, WPI-MANA, NIMS at the time of this project) and Makito Nakatsu (Researcher, RCFM, NIMS at the time of this project).
This research was published in Scientific Reports, an open access journal, on June 9, 2021, Japan Time.
(Regarding this research)
Ryo Tamura
Senior Researcher
First-Principles Simulation Group, MANA, NIMS
Tel:+81-29-860-4948
E-Mail:TAMURA.Ryo=nims.go.jp=nims.go.jp
(Please change "=" to "@")
Kota Shiba
Senior Researcher
Olfactory Sensors Group
Electric and Electronic Materials Field
Research Center for Functional Materials
NIMS
Tel:+81-29-860-4603
E-Mail:SHIBA.Kota=nims.go.jp
(Please change "=" to "@")
Kosuke Minami
ICYS Researcher
Olfactory Sensors Group
International Center for Young Scientists
NIMS
Tel:+81-29-851-3354
E-Mail:MINAMI.Kosuke=nims.go.jp
(Please change "=" to "@")
Genki Yoshikawa
Group Leader
Olfactory Sensors Group
Electric and Electronic Materials Field
Research Center for Functional Materials
NIMS
Tel:+81-29-860-4749
E-Mail:YOSHIKAWA.Genki=nims.go.jp
(Please change "=" to "@")
(General information)
Public Relations Office
National Institute for Materials Science
Tel:+81-29-859-2026
Fax:+81-29-859-2017
E-Mail:pressrelease=ml.nims.go.jp
(Please change "=" to "@")