Joint Workshop LANL/NIMS Quantum and Functional Materials and MANA International Symposium 2024
Quantum Materials - 23
Abstract
In recent years, as high-power consumption by AI has become increasingly critical, there is growing interest in new computing systems inspired by the efficient information processing seen in biological organisms and neural systems. Physical reservoir computing (PRC), which treats the spatiotemporal state evolution of inherent dynamics in materials and devices as a virtual neural network, stands out as a promising approach for bio-inspired computing. We have developed ion-gating reservoirs (IGR) that use the modulation of material properties by ion-gating as a computational resource for PRC. By applying this concept to various material systems, we have successfully demonstrated high-performance information processing and brain function emulation based on dynamics such as the electric double-layer (EDL) effect, redox reactions, and molecular vibration modulation
In this presentation, we will report on the development of IGR across different material systems and provide our latest results on ion-gel/graphene-based IGR (Fig. (a)). In this system, the coexistence of the EDL effect, the bipolar behavior of graphene, and charge trapping dynamics creates complex spatiotemporal state evolutions, expected to lead to brain-like complex network properties. In predicting the Mackey-Glass equation (a chaotic system), which is a widely used benchmark task in machine learning, our IGR is as accurate as deep learning (DL), but has a computational load two orders of magnitude lower than DL. Furthermore, it achieved an extremely high computational efficiency comparable to or even surpassing the theoretical limit of the efficiency estimated from a well-tuned simulated-reservoir computing (RC). This result demonstrates the superior efficiency of IGR and marks a significant step towards realizing bio-inspired computing that emulates the efficient, sophisticated information processing functions found in the brain and biological systems. This research was partially supported by JST PRESTO (JPMJPR23H4) and JSPS KAKENHI (24KJ0229).
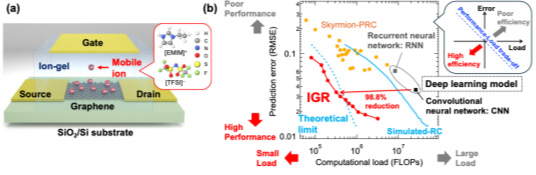
Reference
- D. Nishioka et al., Sci. Adv. 8(15), eade1156, (2022). DOI 10.1126/sciadv.ade1156
- D. Nishioka et al., Neuromorph. Comput. Eng. 3, 034008, (2023). DOI 10.1088/2634-4386/acf1c6
- D. Nishioka et al., Sci. Adv. 10(9), eadk6438, (2024). DOI 10.1126/sciadv.adk6438
- D. Nishioka et al., Commun. Eng. 3, 81, (2024). DOI 10.1038/s44172-024-00227-y