Joint Workshop LANL/NIMS Quantum and Functional Materials and MANA International Symposium 2024
Quantum Materials - 22
Abstract
Physical reservoir computing (PRC) is an artificial neural network in which reservoir computing is performed on physical devices with nonlinear mapping capability to high-dimensional space, and the PRC is attracting attention as edge AI operating on terminal device due to its low learning cost and high processing speed. However, some fatal issues are remained on the road to the implementation as follows; low computational power, high electrical power consumption, and large device volume. In recent years, among such physical devices for the PRC (i.e., physical reservoir), we tracked down that chaotic interfered spin waves in a ferromagnetic material show excellent computing capability as physical reservoir.
Figure (a) shows a schematic illustration of the fabricated iono-magnonic reservoir using two excitation and two detection antennas on a Y3Fe5O12 (YIG) single crystal. Proton (H
This work was partially supported by Innovative Science and Technology Initiative for Security Grant Number JPJ004596, ATLA, Japan, and JSPS KAKENHI Grant Numbers JP22H04625 and JP19H05814 (Grant-in-Aid for Scientific Research on Innovative Areas “Interface Ionics”).
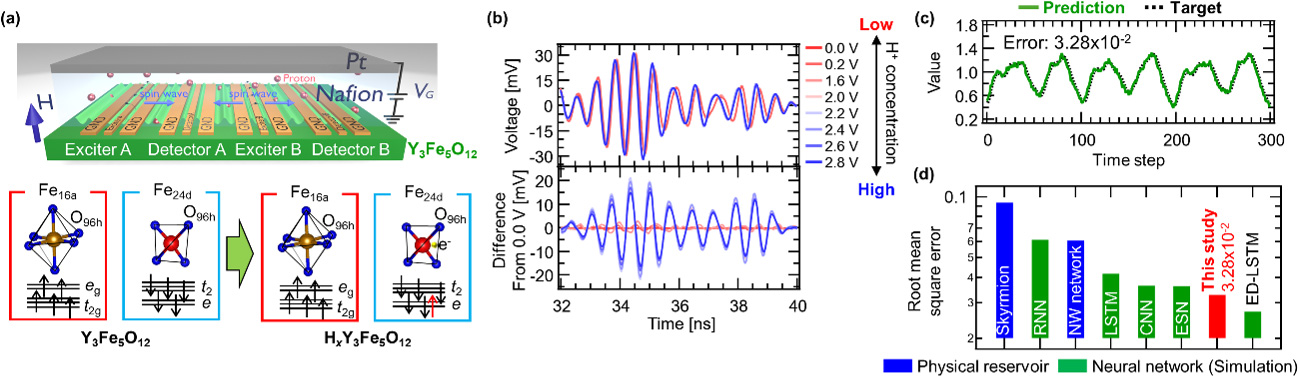
Reference
- W. Namiki et al., Adv. Intell. Syst. 5(12), 2300228, (2023). DOI 10.1002/aisy.202300228
- W. Namiki et al., Mat. Today Phys. 45, 101465 (2024). DOI 10.1016/j.mtphys.2024.101465
- W. Namiki et al., Neuromorphic Comput. and Eng. 4, 024015 (2024). DOI 10.1088/2634-4386/ad561a
- W. Namiki et al., ACS Nano 14(11), 16065-16072 , (2020). DOI 10.1021/acsnano.0c07906
- W. Namiki et al., Adv. Sci. in revision