Joint Workshop LANL/NIMS Quantum and Functional Materials and MANA International Symposium 2024
Quantum Materials - 17
Abstract
Understanding the structural disorders such as phase transitions, atom bombardment, and defects in GaN is of importance aspect in the development of high-quality GaN-based devices. Such process is difficult to observe experimentally due to taking place at extreme pressure and temperature conditions and/or requires such a sophisticated technique as well as involves local structural modification. Capturing this process should be valuable for the characterization of GaN at critical conditions such as a melting point which has been an unresolved problem in the study of GaN
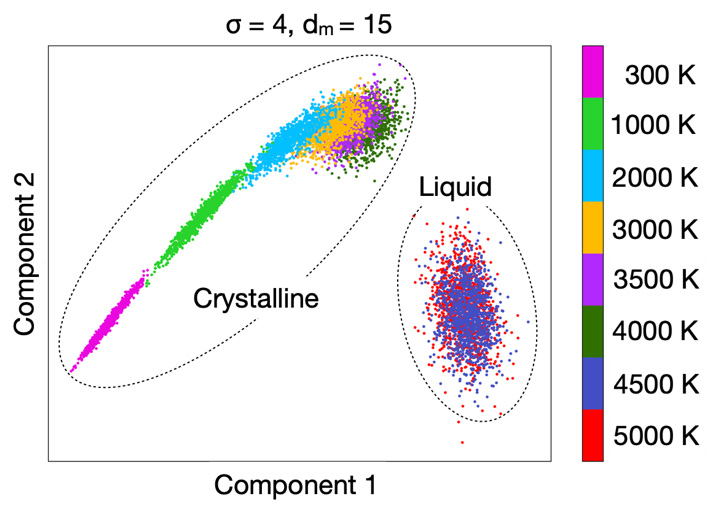
Reference
- S. Porowski, B. Sadovyi, S. Gierlotka, S.J. Rzoska, I. Grzegory, I. Petrusha, V. Turkevich, and D. Stratiichuk, J. Phys. Chem. Solids., 85, 138-143, (2015). DOI 10.1016/j.jpcs.2015.05.006
- R. Tamura, M. Matsuda, J. Lin, Y. Futamura, T. Sakurai, and T. Miyazaki, Phys. Rev. B 105, 075107, (2022). DOI 10.1103/PhysRevB.105.075107
- A. K. A. Lu, J. Lin, Y. Futamura, T. Sakurai, R. Tamura, and T. Miyazaki, Phys. Chem. Chem. Phys. 26, 11657-11666, (2024). DOI 10.1039/d3cp06298h